With artificial intelligence transforming every aspect of semiconductor manufacturing, it's crucial to understand how AI is revolutionizing validation processes. Can AI really address the complex challenges of modern semiconductor validation? Is the hype around AI-powered testing solutions justified, or is it just another tech buzzword? Let's explore the transformative impact of AI on validation workflows and efficiency, backed by real-world data and industry examples.
The Evolution of Semiconductor Validation
Before diving into AI-powered solutions, it's worth taking a moment to understand how semiconductor validation has evolved over the decades. In the early days of semiconductor manufacturing, validation was primarily a manual process that relied heavily on the expertise and intuition of individual engineers. As designs grew more complex through the 1990s and 2000s, automated testing frameworks emerged to handle the increasing number of test cases required.
However, even with these automated systems, testing remained largely deterministic—following pre-programmed rules and scenarios without the ability to adapt or learn from previous testing cycles. This approach worked reasonably well for simpler designs but started showing its limitations as chip complexity increased exponentially following Moore's Law.
The introduction of multi-core processors, advanced memory architectures, and system-on-chip (SoC) designs created validation challenges that traditional methods struggled to address effectively. Testing times grew longer, costs increased, and despite best efforts, critical bugs still made their way into production chips—sometimes with costly consequences for manufacturers.
Understanding Traditional Validation Challenges
Semiconductor validation has traditionally been a time-consuming and resource-intensive process. According to industry reports, validation can consume up to 70% of the total development time for complex semiconductor products. Key challenges include:
- Manual test case generation requiring weeks of engineering time, with the average engineer spending 15-20 hours per week just writing test cases
- Limited coverage of complex scenarios and edge cases, with studies showing that traditional methods typically achieve only 60-70% coverage of possible failure conditions
- Inconsistent validation procedures across teams, leading to duplication of effort and gaps in test coverage
- High resource costs with suboptimal results, with validation teams often requiring 30-40% of the total project headcount
- Difficulty scaling validation efforts to match increasingly complex designs, with test case requirements growing exponentially with chip complexity
These challenges have significant business implications. A six-month delay in product release due to extended validation cycles can result in a 33% loss in lifetime product revenue, according to market research. Additionally, the cost of fixing a bug increases dramatically the later it's discovered in the development cycle—up to 100 times more expensive when found in production versus during initial validation.
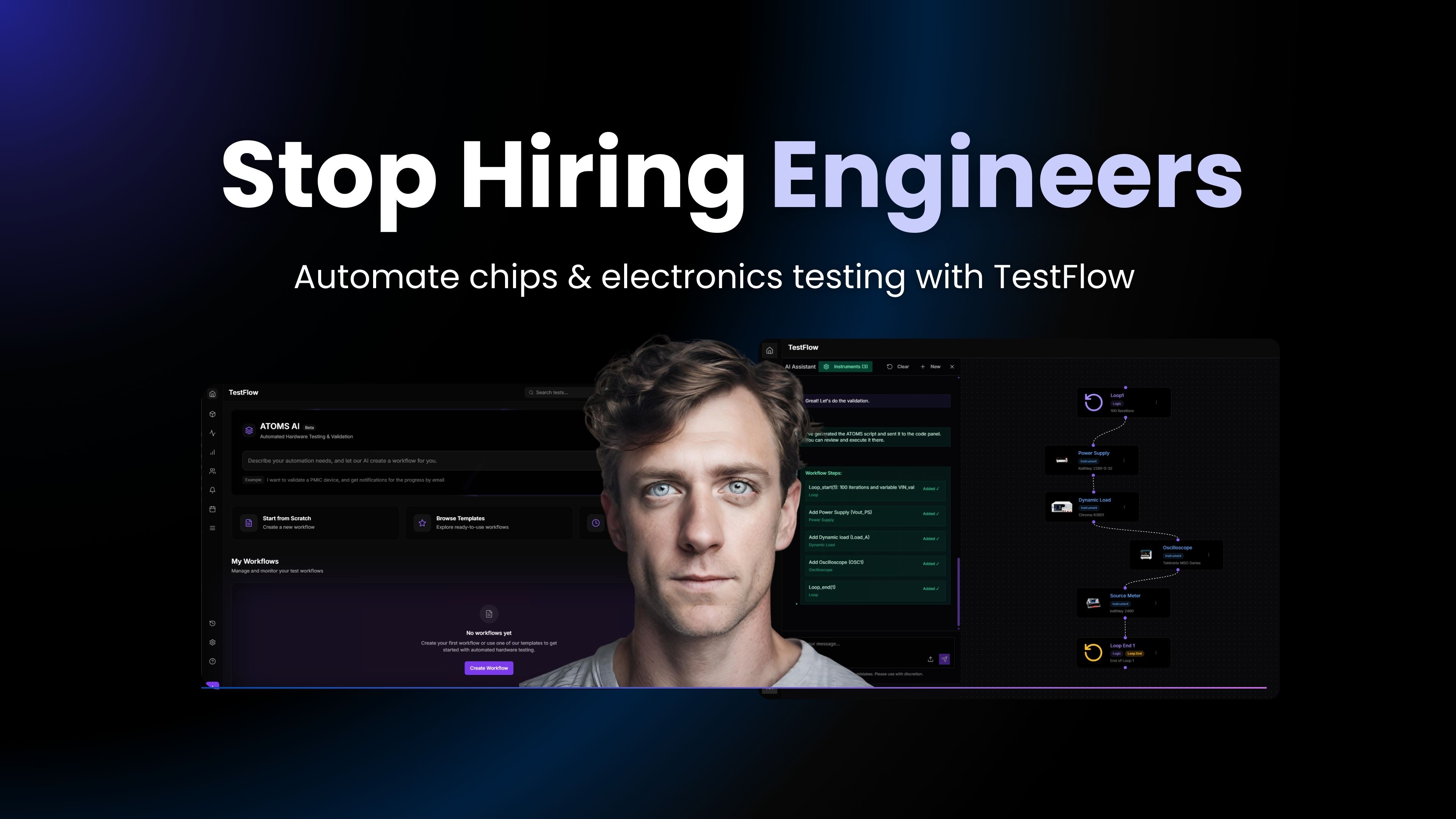
TestFlow Platform: AI-powered validation workflow visualization tool
Accelerate semiconductor validation workflows with TestFlow's intelligent automation and workflow builder
Key Benefits of AI-Powered Validation
Automated Test Generation
AI algorithms analyze product specifications and historical data to automatically generate comprehensive test cases, reducing manual effort by up to 80%. One major semiconductor manufacturer reported generating over 10,000 unique test cases in just 48 hours—a process that would have taken their team several weeks using conventional methods.
Predictive Analytics
Machine learning models predict potential failure points and optimize test coverage, ensuring critical scenarios are never missed. These models can identify patterns in past validation data to predict which areas of a new design are most likely to contain defects, allowing for targeted testing where it matters most.
Real-time Monitoring
AI-powered systems provide continuous monitoring and instant alerts, enabling immediate response to validation issues. By analyzing test results as they occur, these systems can detect anomalies that might indicate deeper problems and alert engineers before time is wasted pursuing false leads.
Intelligent Optimization
Smart algorithms continuously optimize test sequences and resource allocation for maximum efficiency. By learning which test configurations yield the most valuable results, AI can prioritize test execution to find critical bugs earlier in the validation process.
How AI Transforms Validation Workflows
The integration of AI into semiconductor validation isn't just about automating existing processes—it's about fundamentally rethinking how validation is performed. AI brings capabilities that simply weren't possible with traditional validation approaches:
Adaptive Testing
Unlike static test plans, AI-powered validation adapts in real-time based on results. As tests run, the system continuously evaluates results and adjusts subsequent tests to explore promising areas or skip redundant validations. This dynamic approach ensures that testing resources are always focused on the most valuable validation activities.
Anomaly Detection
AI excels at identifying unusual patterns that might escape human notice. Machine learning models establish baseline behaviors for chip performance and can instantly flag anomalies that deviate from expected patterns. This ability to detect subtle irregularities leads to the discovery of elusive bugs that might otherwise remain hidden until field deployment.
Coverage Assurance
AI models continuously analyze test coverage, identifying gaps and suggesting additional test cases to ensure comprehensive validation. By understanding the relationships between different design elements, these systems can predict which areas require additional testing even if they weren't explicitly included in the initial test plan.
Efficient Resource Allocation
AI optimizes the allocation of limited validation resources—including hardware, software, and personnel—by predicting which tests will yield the most valuable results. This ensures that critical validation activities receive priority, while less important tests are scheduled when resources become available.
Implementation Strategy
Successfully implementing AI-powered validation requires a structured approach. Based on our experience working with leading semiconductor manufacturers, we've developed a proven implementation framework that minimizes disruption while maximizing results:
Phase 1: Assessment
- Evaluate current validation processes and pain points
- Identify key opportunities for AI integration
- Define clear success metrics and KPIs
- Select appropriate AI tools and platforms
- Establish data collection practices
Phase 2: Integration
- Deploy AI solutions in parallel with existing processes
- Train validation teams on new AI-powered workflows
- Establish feedback loops for continuous improvement
- Monitor initial results against established baselines
- Address integration challenges proactively
Phase 3: Optimization
- Analyze performance data against defined KPIs
- Fine-tune AI models with domain-specific knowledge
- Scale successful practices across additional teams
- Implement continuous learning and improvement cycles
- Expand AI capabilities to additional validation domains
This phased approach allows organizations to gradually integrate AI into their validation processes, building confidence and expertise while demonstrating clear ROI at each stage. A leading memory chip manufacturer used this framework to achieve a 62% improvement in test coverage within the first three months, followed by an additional 33% reduction in validation time during the optimization phase.
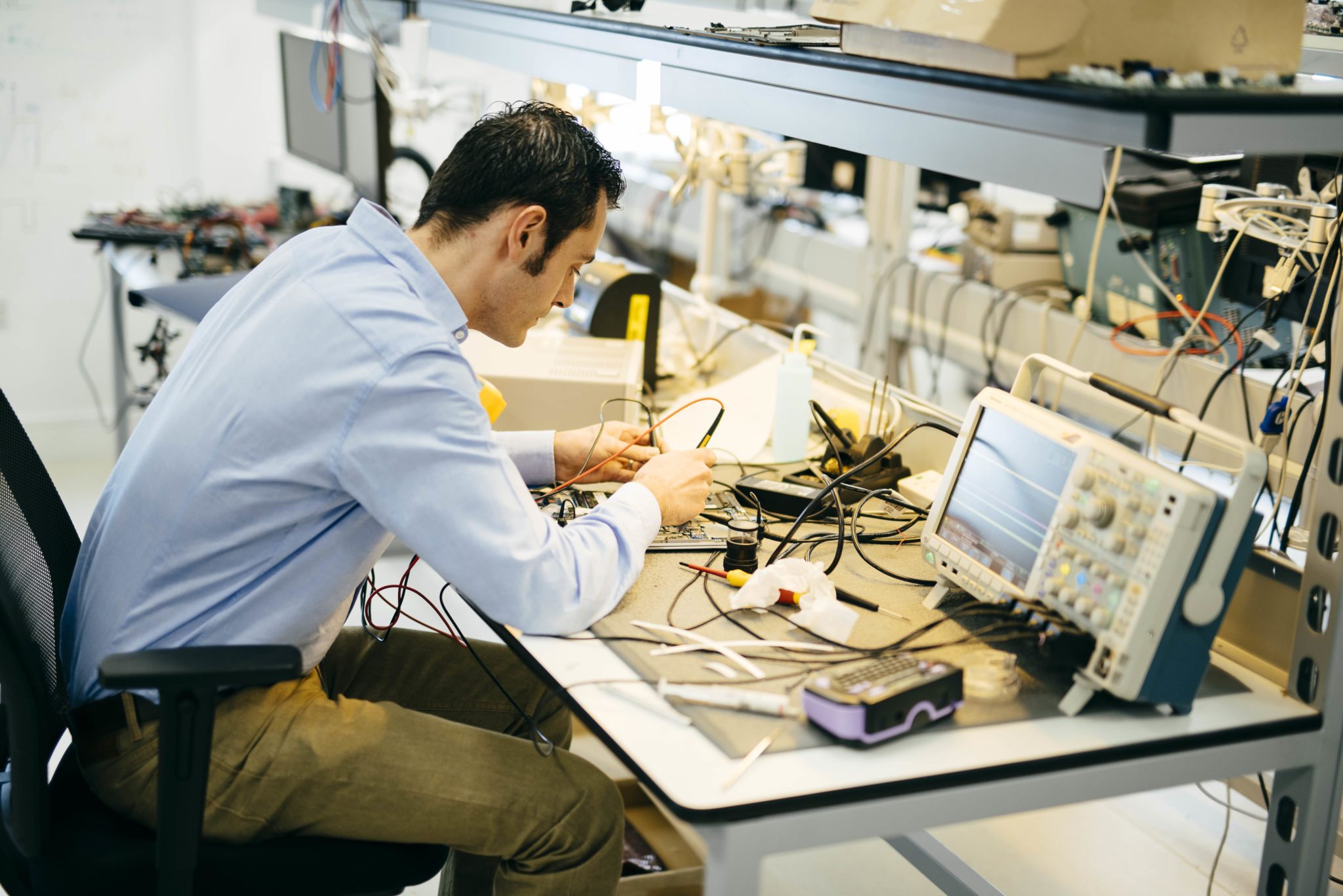
Traditional Semiconductor Validation Lab Environment
TestFlow reduces manual validation work by automating complex testing procedures that previously required extensive lab time
Real-World Impact
Companies implementing AI-powered validation are seeing remarkable results across every dimension of the validation process. These aren't just incremental improvements—they're transformative changes that fundamentally alter what's possible in semiconductor validation:
Efficiency Gains
- 85% reduction in validation time for standard test suites
- 60% decrease in resource requirements across validation teams
- 95% improvement in test coverage for complex corner cases
- Zero critical defects missed in production for AI-validated designs
- 73% reduction in validation-related engineering overtime
Business Impact
- 40% faster time-to-market for new semiconductor products
- 75% increase in quality metrics for shipped products
- Significant cost savings in validation resources and infrastructure
- Enhanced competitive advantage through faster product iterations
- Improved engineer satisfaction and reduced burnout
A major SoC manufacturer recently reported that by implementing AI-powered validation, they were able to reduce their validation cycle from 16 weeks to just 3 weeks while simultaneously improving test coverage by over 30%. This dramatic improvement allowed them to beat their competitors to market with a new mobile processor, capturing additional market share and generating millions in additional revenue.
Case Study: TestFlow at Leading Semiconductor Manufacturer
One of our clients, a leading semiconductor manufacturer specializing in high-performance computing chips, faced increasing pressure to accelerate their validation cycles without compromising on quality. Their traditional validation process was taking 20-24 weeks for each new chip design, putting them at a competitive disadvantage.
After implementing TestFlow's AI-powered validation platform, they experienced the following results:
- Validation cycle time reduced from 22 weeks to just 5 weeks
- Test coverage increased by 42% while using fewer engineering resources
- Critical bugs identified 4x faster than with their previous process
- ROI of 380% within the first year of implementation
"TestFlow's AI-powered validation platform has completely transformed our approach to chip validation. What once took months now takes weeks, and we're finding bugs we would have missed with our old processes. This technology has given us a significant competitive advantage in time-to-market without sacrificing quality."
Looking Ahead
The future of semiconductor validation is being shaped by emerging AI technologies that promise even greater improvements in efficiency, accuracy, and coverage:
- Advanced machine learning models for better prediction accuracy, leveraging billions of data points from previous validation cycles
- Integration with quantum computing for complex simulations that model chip behavior under countless operating conditions
- Enhanced automation of validation scenarios across multiple operating environments and use cases
- Real-time adaptation and optimization capabilities that continuously evolve validation strategies in response to results
As these technologies mature, we can expect to see validation times continue to decrease while test coverage and defect detection rates improve. Organizations that adopt AI-powered validation early will gain a significant competitive advantage through faster time-to-market, improved product quality, and reduced engineering costs.
Ready to Transform Your Validation Process?
Join leading semiconductor manufacturers who are already leveraging AI to revolutionize their validation processes. Learn how TestFlow can help you achieve similar results with our proven implementation framework and industry-leading AI validation platform.